Dear friends,
This week, I’m speaking at the World Economic Forum (WEF) and Asia-Pacific Economic Cooperation (APEC) meetings in San Francisco, where leaders in business and government have convened to discuss AI and other topics. My message at both events is simple: Governments should not outlaw open source software or pass regulations that stifle open source development.
Regulating AI is a hot topic right now in the United States, European Union, and elsewhere. Just this week, the EU’s AI Act was derailed when France and Germany objected — with good reason, in my view — to provisions that would burden companies that build foundation models.
As Yann LeCun and I have said, it’s important to distinguish between regulating technology (such a foundation model trained by a team of engineers) and applications (such as a website that uses a foundation model to offer a chat service, or a medical device that uses a foundation model to interacts with patients). We need good regulations to govern AI applications, but ill-advised proposals to regulate the technology would slow down AI development unnecessarily. While the EU’s AI Act thoughtfully addresses a number of AI applications — such as ones that sort job applications or predict crime — and assesses their risks and mandates mitigations, it imposes onerous reporting requirements on companies that develop foundation models, including organizations that aim to release open-source code.
I wrote in an earlier letter that some companies that would rather not compete with open-source, as well as some nonprofits and individuals, are exaggerating AI risks. This creates cover for legislators to pass regulations in the name of safety that will hamper open source. At WEF and APEC, I’ve had conversations about additional forces at play. Let me describe what I’m seeing.
In the U.S., a faction is worried about the nation’s perceived adversaries using open source technology for military or economic advantage. This faction is willing to slow down availability of open source to deny adversaries’ access. I, too, would hate to see open source used to wage unjust wars. But the price of slowing down AI progress is too high. AI is a general-purpose technology, and its beneficial uses — similar to other general purpose technologies like electricity — far outstrip the nefarious ones. Slowing it down would be a loss for humanity.
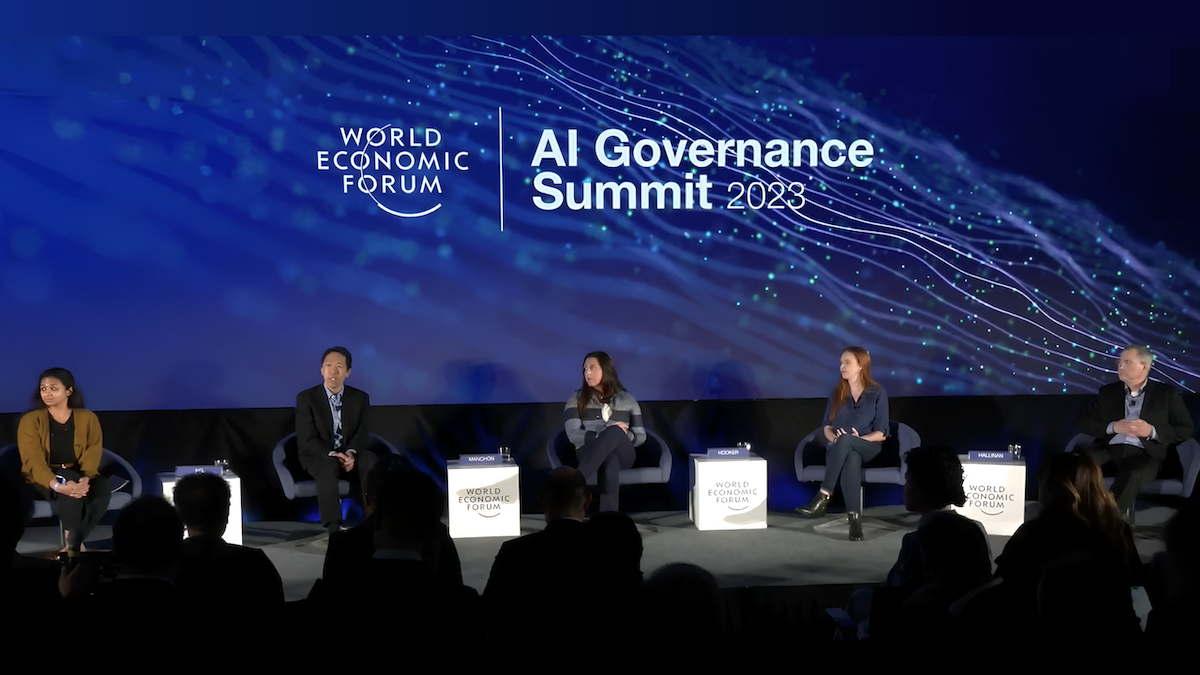
When I speak with senior U.S. government officials, I sense that few think the possibility that AI will lead to human extinction is a realistic risk. This topic tends to lead to eye-rolls. But they genuinely worry about AI risks such as disinformation. In comparison, the EU is more concerned — unnecessarily, in my view — about the risk of extinction, while also worried about other, more concrete harms.
Many nations and corporations are coming to realize they will be left behind if regulation stifles open source. After all, the U.S. has a significant concentration of generative AI talent and technology. If we raise the barriers to open source and slow down the dissemination of AI software, it will only become harder for other nations to catch up. Thus, while some might argue that the U.S. should slow down dissemination of AI (an argument that I disagree with), that certainly would not be in the interest of most nations.
I believe deeply that the world is better off with more intelligence, whether human intelligence or artificial intelligence. Yes, intelligence can be used for nefarious purposes. But as society has developed over centuries and we have become smarter, humanity has become much better off.
A year ago, I wouldn’t have thought that so many of us would have to spend so much time trying to convince governments not to outlaw, or make impractical, open-sourcing of advanced AI technology. But I hope we can all keep on pushing forward on this mission, and keep on pushing to make sure this wonderful technology is accessible to all.
Keep learning!
Andrew
P.S. Many teams that build applications based on large language models (LLMs) worry about their safety and security, and such worries are a significant barrier to shipping products. For example, might the application leak sensitive data, or be tricked into generating inappropriate outputs? Our new short course shows how you can mitigate hallucinations, data leakage, and jailbreaks. Learn more in “Quality and Safety for LLM Applications,” taught by Bernease Herman and created in collaboration with WhyLabs (disclosure: an AI Fund portfolio company). Available now!
News
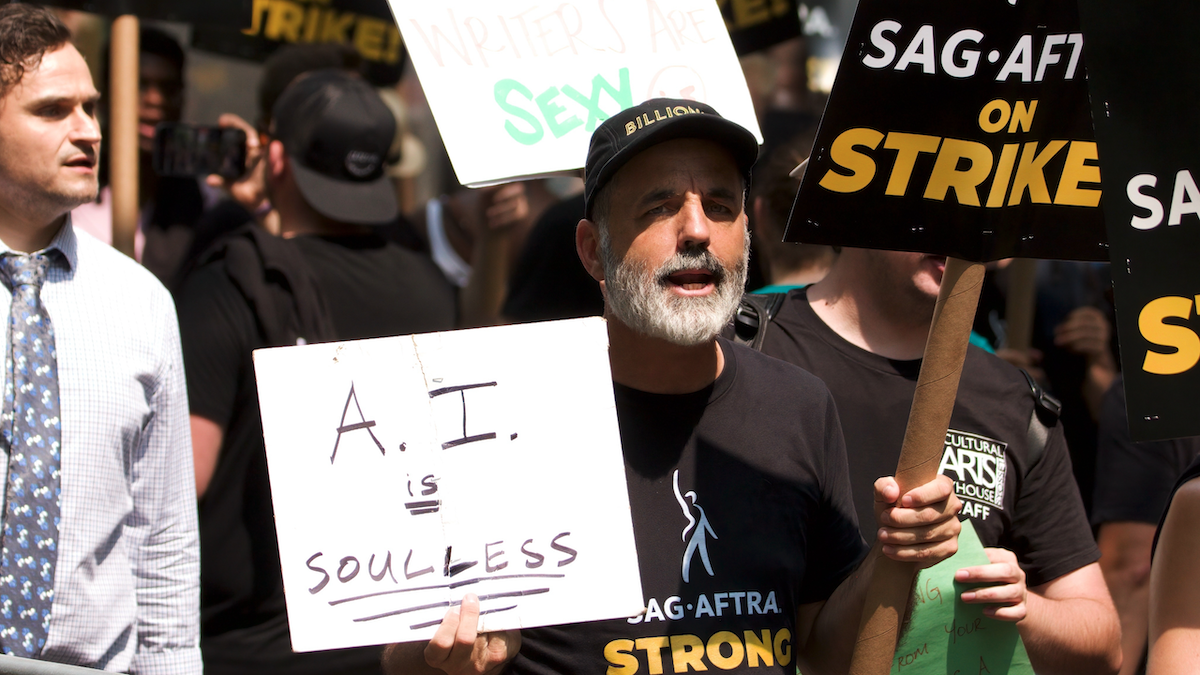
Actors Reach Accord on AI
The longest actors’ strike in Hollywood history ended as actors and studios reached an accord on the use of generative AI in making movies.
What’s new: Film studios must seek an actor’s consent before using a generated likeness or performance and compensate the actor, according to an agreement between the trade union Screen Actors Guild-American Federation of Television and Radio Artists (SAG-AFTRA) and the Alliance of Motion Picture and Television Producers (TMPTP). The pact will remain in effect for three years, once it has been ratified by SAG-AFTRA members.
How it works: The agreement covers digital replicas of human actors, synthetic performers, and simulated performances created using AI and other technologies that may not be generally recognized as AI. The parties argued over terms with respect to AI until the very last day of their 118-day negotiation, according to SAG-AFTRA’s president. Among the provisions:
- Studios must compensate an actor if performances are used to train a model.
- Studios must secure an actor’s consent before using a synthetic likeness or performance, regardless of whether the replica was made by scanning the actor or extracting information from existing footage. The actor has the right to refuse. If the actor consents, studios must compensate the actor for the days they would have worked, if they had performed in person.
- Studios may use digital replicas of recognizable actors who have background roles and don’t speak, but they must compensate the actors. If studios alter a synthetic background actor so it appears to speak, they must pay the actor a full wage.
- If studios want to synthesize a deceased actor who did not consent while alive, they must seek consent from the heirs or estate.
- Studios can combine the likenesses of multiple actors into a “synthetic performer,” but they must seek consent and compensate the actors for “recognizable elements” they use. In addition, they must notify SAG-AFTRA and allow the union to bargain on behalf of the actors.
- TMPTP must meet with SAG-AFTRA semi-annually to review the state of affairs in AI, giving the actors an opportunity to adjust guidelines in response as technology and law develop.
Behind the news: The agreement followed a similar three-year deal in September that ended the concurrent strike by Writers Guild of America.
Yes, but: The agreement covers on-screen actors. It does not cover voice or motion actors in video games or television animation. In September, SAG-AFTRA authorized a strike against a group of video game companies if negotiations, which are ongoing, stall. Negotiations over television animation are expected as well.
Why it matters: The actors’ agreement could set an international example for limits on AI in the performing arts, thanks to the U.S. film and television industry’s global reach. Entertainers’ unions in Europe and Canada are contemplating strikes inspired by SAG-AFTRA’s, and they may seek similar agreements.
We’re thinking: As with the screenwriters’ contract, the agreement between actors and studios gives everyone three years to experiment with AI while respecting the consent, credit, and compensation of creative workers. We hope that shows made in this period provide ample evidence that such tools can yield wonderful productions that enlarge the market, and that the next agreement focuses more on growing the use of AI and dividing the winnings fairly among actors, studios, and technologists.
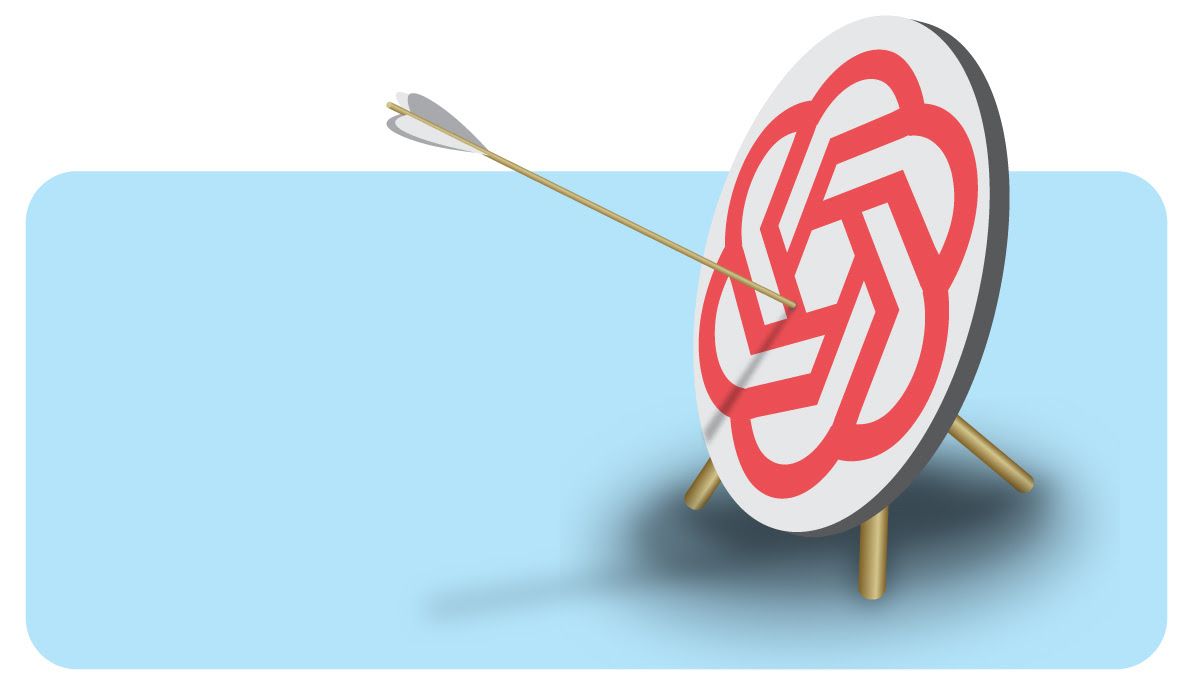
Cyberattack Strikes OpenAI
ChatGPT suffered a cyberattack apparently tied to the Kremlin.
What's new: A ChatGPT outage on November 8 most likely was caused by a distributed denial of service (DDoS) attack, OpenAI revealed.
What happened: ChatGPT went down shortly before 9:00 a.m. Eastern Time and remained out of service for about 90 minutes. Intermittent outages of unknown cause had affected OpenAI and other services during the previous two days.
- Initially, OpenAI CEO Sam Altman claimed the outages reflected high user interest after OpenAI had announced new features earlier in the week. Later, the company stated that the traffic pattern suggested malicious activity consistent with DDoS.
- A group called Anonymous Sudan claimed responsibility. Anonymous Sudan has been linked to previous cyberattacks on Microsoft, X, NATO, the European Investment Bank, and a number of Israeli civilian and military institutions. The group purports to operate from Africa on behalf of oppressed Muslims around the world, but some cybersecurity analysts believe it’s linked to the Russian government.
- The outage followed less-critical incidents during the prior two days; the causes have not been reported. On November 8, DALL·E 3’s API showed elevated error rates throughout the day. The previous day, parts of OpenAI’s API were unavailable at times.
- ChatGPT competitor Claude 2 also reported services issues on November 8 due to an unknown cause.
DDoS basics: In a DDoS attack, malicious programs running independently on numerous machines flood a website with requests, disrupting service. The distributed nature of the attack makes it difficult to trace or combat. Almost all cloud providers and large websites use DDoS mitigation services or their own technology to defend against such attacks. However, such defenses don’t always block an especially determined or resourceful attacker.
Why it matters: The ChatGPT outage is a sobering reminder that API-powered services are vulnerable to targeted attacks, and providers need to be proactive about protecting themselves and their users.
We're thinking: While no one likes downtime, it’s hard to defend against a state-sponsored DDoS. It’s a testament to OpenAI’s impact that just 90 minutes of downtime was felt around the world.
A MESSAGE FROM DEEPLEARNING.AI
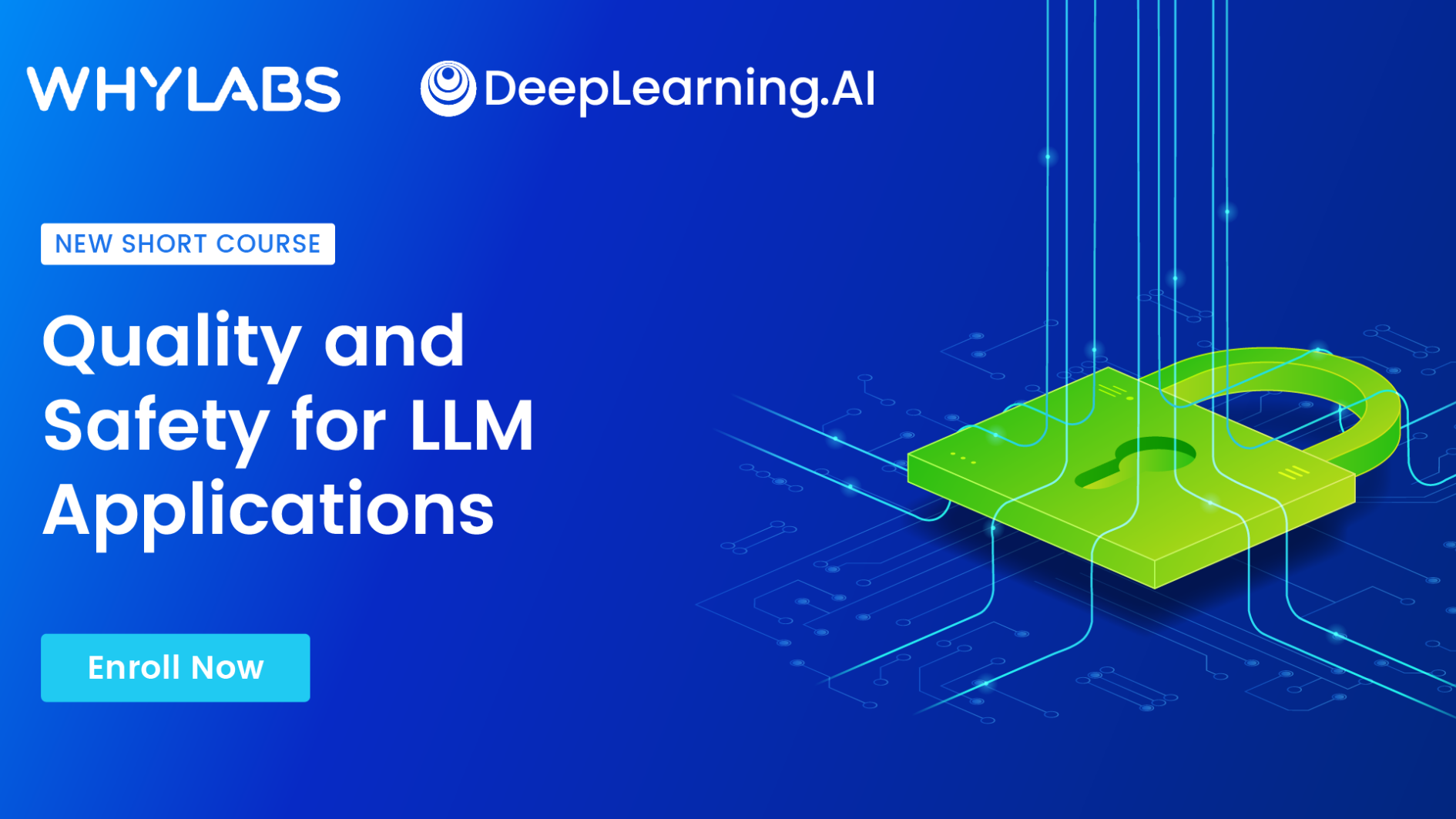
New short course! “Quality and Safety for LLM Applications” will help you enhance the safety of large language model applications by detecting issues like data leakage, hallucination, toxicity, and jailbreaks. Start making your apps more secure today. Enroll now
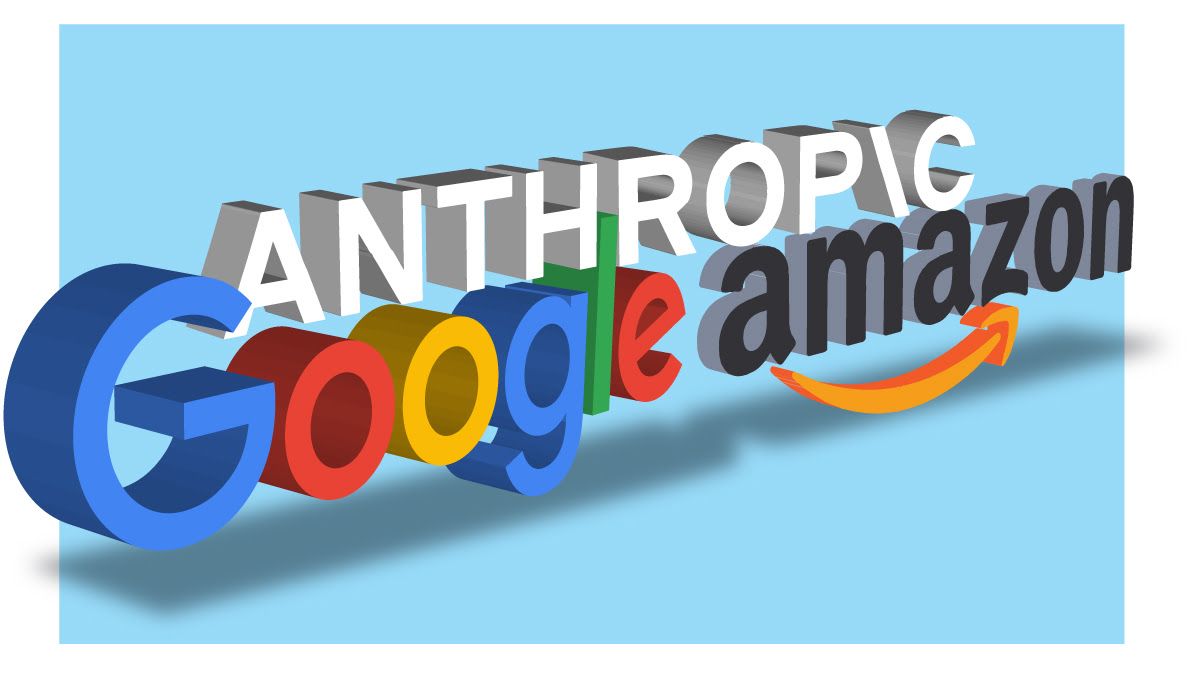
Anthropic Cultivates Alternatives
Weeks after it announced a huge partnership deal with Amazon, Anthropic doubled down on its earlier relationship with Alphabet.
What's new: Anthropic, which provides large language models, agreed to use Google’s cloud-computing infrastructure in return for a $2 billion investment, The Wall Street Journal reported. The deal follows an earlier multibillion-dollar partnership that saw Anthropic commit to training new models on Amazon Web Services.
How it works: Google invested $500 million up front and will add $1.5 billion more over an unspecified time period. The new funding builds on $300 million that Google gave to Anthropic earlier in the year for a 10 percent stake in the company. Google’s current stake in Anthropic is undisclosed.
- Anthropic agreed to spend $3 billion on Google Cloud over four years. Anthropic will use Google’s newly available TPU v5e AI processors to scale its Claude 2 large language model for cloud customers. However, it will continue to run most of its processing on Amazon hardware.
- The startup will use Google’s AlloyDB database to handle accounting data and BigQuery for data analysis.
- Google Cloud CEO Thomas Kurian said Google will draw on Anthropic’s experience in AI safety techniques such as constitutional AI, a method for training large language models to behave according to a set of social values.
Behind the news: Anthropic rose rapidly from AI startup to coveted foundation-model partner.
- Anthropic was founded by former OpenAI engineers who left that company, believing that it had abandoned its original principles. Early on, the startup received $500 million from cryptocurrency exchange FTX. When FTX collapsed less than a year ago, Anthropic worried that creditors might claw back the funds.
- In March, Anthropic introduced Claude, a large language model trained via constitutional AI. Claude 2 followed in July.
- Last month, Anthropic sealed a $4 billion investment from Amazon, giving the retail giant a minority stake. The startup committed to using Amazon chips to train its models, while Amazon will receive special access to Claude 2 and other Anthropic models to train its own generative models. Amazon is developing a 2 trillion-parameter model codenamed Olympus that will encompass 2 trillion parameters, 14 times the size of Claude 2.
Why it matters: The Anthropic-Google deal changes the shape of the startup’s relationships with large cloud providers. Anthropic's deal with Amazon dwarfed Google’s initial investment and seemed like a formative partnership akin to OpenAI’s lucrative Microsoft pair-up. Now, Anthropic is more like a vertex in a triangle, bound by close relationships with competing partners.
We're thinking: Anthropic hasn’t raised as much total funding as OpenAI ($12.7 billion and counting), but its relationships with both Google and Amazon give it more flexibility to choose different infrastructure for different tasks. The benefits presumably will flow not only to the three companies but also to independent developers, who can choose among stellar proprietary foundational models — not to mention open source alternatives — from three major cloud providers.
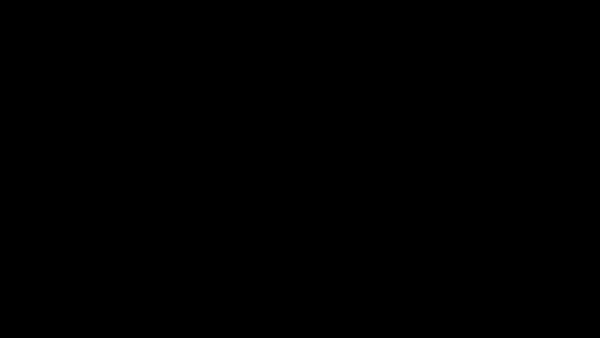
AI Builds Better Sorting Algorithms
Online sorting algorithms run trillions of times a day to organize lists according to users’ interests. New work found faster alternatives.
What’s new: Daniel J. Mankowitz and colleagues at Google developed AlphaDev, a system that learned to generate algorithms that sort three to five numbers faster than previous state-of-the-art methods. Accelerating such algorithms can expedite the sorting of lists of any size — say, for search engines, ecommerce sites, and the like — since algorithms that sort more elements often call algorithms that sort fewer elements.
Key insight: Most programmers implement sorting algorithms in a high-level programming language like C++, which a compiler translates into Assembly Language instructions that control the processor and memory. A compiler can translate a single line of C++ into a variety of sequences of Assembly instructions that are equivalent functionally but vary in their speed (number of Assembly instructions required). A reinforcement learning agent can learn to choose a translation that maximizes speed.
How it works: AlphaDev is a collection of neural networks that learn jointly via reinforcement learning. The authors initialized the system by giving it a sequence of unsorted numbers and an empty list of Assembly instructions. It built algorithms by adding Assembly instructions one by one. It earned rewards for choosing instructions that sorted the numbers correctly and quickly.
- With each new instruction selected, a transformer computed an embedding of the instructions so far, and a vanilla neural network computed an embedding of the order of the numbers after applying those instructions. The system concatenated the two embeddings to represent the current state.
- Given the embeddings, two vanilla neural networks selected instructions. The first network (i) predicted the total future reward for the current state and (ii) calculated the probability that any given instruction would improve the algorithm. The second network (iii) predicted the reward after adding each possible instruction and (iv) predicted an embedding to represent the resulting state.
- The system searched through possible sequences of instructions to find which instruction most often led to the highest predicted rewards. It added that instruction to the algorithm.
- Once the system had built an algorithm, the authors uploaded it to the main C++ library, which had not been updated in over a decade. The resulting algorithms now serve as open source subroutines in C++’s default sorting algorithm.
Results: The authors tested two approaches to rewarding speed, minimizing either Assembly instructions or average runtime over a number of inputs. When AlphaDev minimized the number of Assembly instructions, it found an algorithm that sorted three integers using 17 instructions instead of the previous state-of-the-art algorithm, a human-engineered one that used 18 instructions. Its algorithm for sorting four integers used 28 instructions, equal to the typical one. Its algorithm for sorting five integers had 42 instructions, compared to the alternative’s 46 instructions. When AlphaDev optimized for runtime (running on Intel 6th-generation Core “Skylake” processor), sorting three integers took 2.18 nanoseconds, compared to the typical algorithm’s 4.86 nanoseconds. Sorting four unsigned integers took 1.96 nanoseconds instead of 5.43 nanoseconds and sorting five of them took 1.98 nanoseconds instead of 6.79 nanoseconds. AlphaDev achieved smaller speedups with longer number sequences: Sorting 16 unsigned integers took 9.5 nanoseconds instead of 10.5 nanoseconds, and sorting 262,144 numbers took 60.8 nanoseconds instead of 61.4 nanoseconds.
Why it matters: This work repurposes the training method and architecture of game-playing models like AlphaZero to solve real-world problems. The trick is to reframe the task of writing a sorting algorithm as a reinforcement learning problem.
We’re thinking: What other algorithms can this approach optimize? How much faster will they be? Let’s get these questions sorted!
A MESSAGE FROM DEEPLEARNING.AI
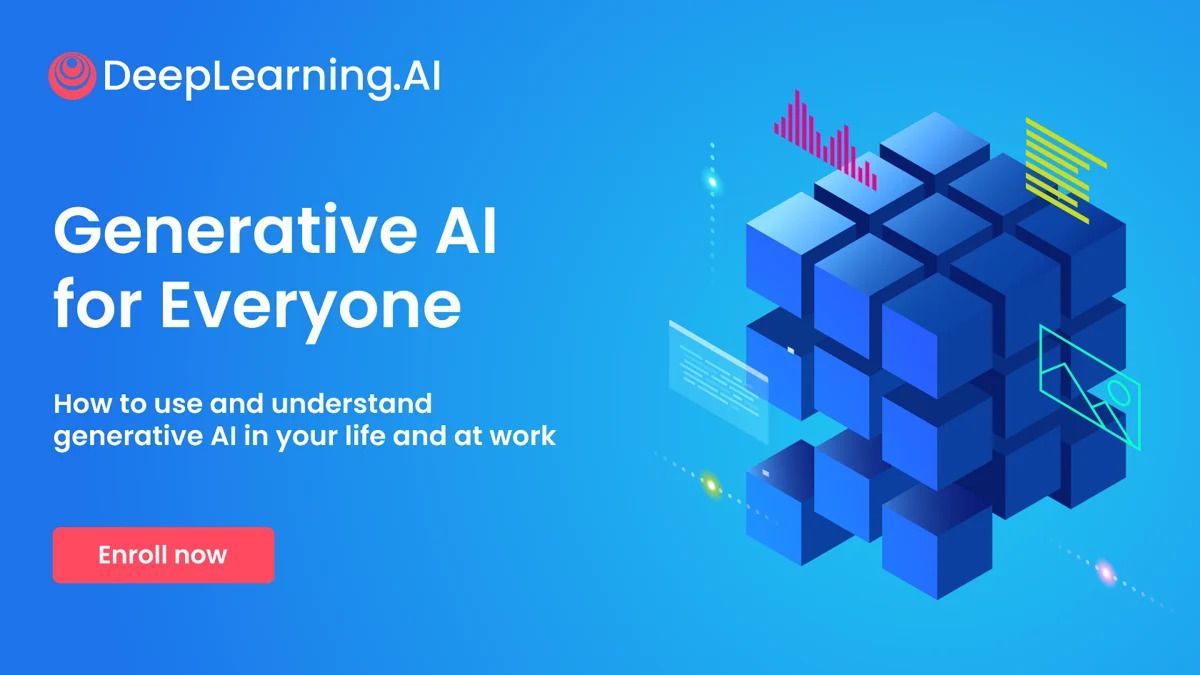
Experience the fastest-growing course on Coursera this year, Generative AI for Everyone! Led by Andrew Ng, delve into generative AI and its applications in both professional and personal settings. Enroll now
Data Points
Start-ups shell out big bucks for AI domain names
As the AI industry continues to boom, entrepreneurs are finding that securing a memorable domain name comes at a hefty price. Domain brokerages report a significant increase in sales for websites with a .ai suffix, with some speculators profiting by flipping domain names. (BBC)
Samsung unveils “Gauss” generative AI model, set to debut in Galaxy S24 series
The model includes language, coding assistant, and image generation sub-models. Samsung's move reflects a broader strategy to apply generative AI across multiple products, with a focus on delivering meaningful and personalized interactions for users. (Korean Times)
Adobe’s generated images of Israel-Hamas conflict slipped into news stories
Adobe's stock image library is under scrutiny as AI-generated images depicting the Israel-Hamas conflict are being sold and subsequently used by news publishers as authentic representations. Despite being labeled as "generated by AI" in Adobe Stock, these images are often presented without disclosure when used in news articles. (The Register)
Meta restricts political advertisers from using generative AI
The decision, revealed in updates in Meta's help center, aims to prevent misuse that could amplify election misinformation. Advertisers dealing with Housing, Employment, Credit, Social Issues, Elections, and sectors like Health, Pharmaceuticals, and Financial Services are currently barred from employing generative AI features. Other tech giants like Google have also implemented similar measures. (Reuters)
Amazon invests millions in training massive AI model "Olympus"
According to insiders, Olympus rivals top models developed by OpenAI and Alphabet. The ambitious project is speculated to possess a staggering 2 trillion parameters, potentially surpassing OpenAI's trillion-parameter GPT-4. While Amazon has trained smaller models like Titan, the development of Olympus underscores the company's commitment to advancing large-scale AI capabilities despite the associated computational challenges. (Reuters)
Microsoft introduces AI characters and stories to Xbox games
An extensive partnership with Inworld AI involves Microsoft’s creation of an AI design copilot system, enabling Xbox developers to craft intricate scripts, dialogue trees, and quest lines. This initiative combines Inworld's expertise in character development with Microsoft's cloud-based AI solutions, including Azure OpenAI Service and technical insights from Microsoft Research. (The Verge)
Research: Nvidia’s ChipNeMo: A custom chip design model trained on internal data
Researchers demonstrated how generative AI, trained on internal data, can serve as a valuable assistant in the process of designing complex chips. The authors envision applying generative AI to various stages of chip design, anticipating substantial gains in overall productivity in the semiconductor industry. The customizable nature of ChipNeMo, with as few as 13 billion parameters, offers superior performance compared to larger general-purpose LLMs, marking an advancement in the application of generative AI to semiconductor engineering. (Nvidia)
GitHub’s Copilot Enterprise enables customization for developers working with internal code
Priced at $39 per person per month, Copilot Enterprise allows customization and tuning for proprietary codebases, addressing the needs of clients with unique programming languages. This move follows Amazon's October announcement that it would offer customization of its CodeWhisperer programming assistant. (CNBC)
Cruise initiates nationwide recall of 950 driverless cars after pedestrian incident
The autonomous vehicle company voluntarily recalled 950 of its driverless cars across the U.S. following a severe crash last month where a pedestrian was hit and dragged for about 20 feet. The recall aims to address a programming flaw related to the "Collision Detection Subsystem," specifically focusing on the post-collision response to prevent similar incidents. Cruise is also considering potential layoffs as it works to rebuild public trust in the aftermath of the incident. (The Washington Post)
Nations agree to set guardrails for military AI
31 nations, including the U.S., signed a nonbinding declaration to establish voluntary guardrails on military AI. The agreement aims to ensure that the development, testing, and deployment of AI in military systems adheres to international laws, promotes transparency, avoids unintended biases, and includes safeguards allowing disengagement in case of unintended behavior. The signatories plan to meet in early 2024 for further discussions. (Wired)
China sets ambitious goal for advanced humanoid robots
The Chinese Ministry of Industry and Information Technology outlined plans for China to produce its first humanoid robots by 2025. The government aims to support young companies in the robotics field, set industry standards, foster talent, and enhance international cooperation. The government's goals include breakthroughs in environment sensing, motion control, and machine-to-human interaction capabilities within the next two years, with plans for humanoid robots to think, learn, and innovate by 2027. (Bloomberg)
Google expands and updates Generative AI in Search to over 120 new countries
The expansion introduces support for four additional languages: Spanish, Portuguese, Korean, and Indonesian. Google also launched upgrades for the U.S. audience, including features such as easier follow-up questions, AI-powered translation assistance, and expanded definitions for topics like coding. (Google)