Dear friends,
I spent Sunday through Tuesday at the CVPR computer vision conference in Vancouver, Canada, along with over 4,000 other attendees. With the easing of the pandemic, it’s fantastic that large conferences are being held in person again!
There’s a lot of energy in computer vision right now. As I recall, the natural language processing community was buzzing about transformers a couple of years before ChatGPT revolutionized the field more publicly. At CVPR, I sensed similar excitement in the air with respect to computer vision. It feels like major breakthroughs are coming.
It is impossible to summarize hundreds of papers into a single letter, but I want to share some trends that I’m excited about:
Vision transformers: The Batch has covered vision transformers extensively, and it feels like they’re still gaining momentum. The vision transformer paper was published in 2020, and already this architecture has become a solid alternative to the convolutional neural network. There are complexities still to be worked out, however. For example, whereas turning a piece of text into a sequence of tokens is relatively straightforward, many decisions need to be made (such as splitting an image into patches, masking, and so on) to turn an image processing problem into a token prediction problem. Many researchers are exploring different alternatives.
Image generation: Algorithms for generating images have been a growing part of CVPR since the emergence of GANs and then diffusion models. This year, I saw a lot of creative work on editing images and giving users more fine-grained control over what such models generate. I also saw a lot of work on generating faces, which is not surprising, since faces interest people.
NeRF: This approach to generating a 3D scene from a set of 2D images has been taking off for a while (and also covered extensively in The Batch). Still, I was surprised at the large number of papers on NeRF. Researchers are working to scale up NeRF to larger scenes, make it run more efficiently, handle moving scenes, work with a smaller number of input images, and so on.
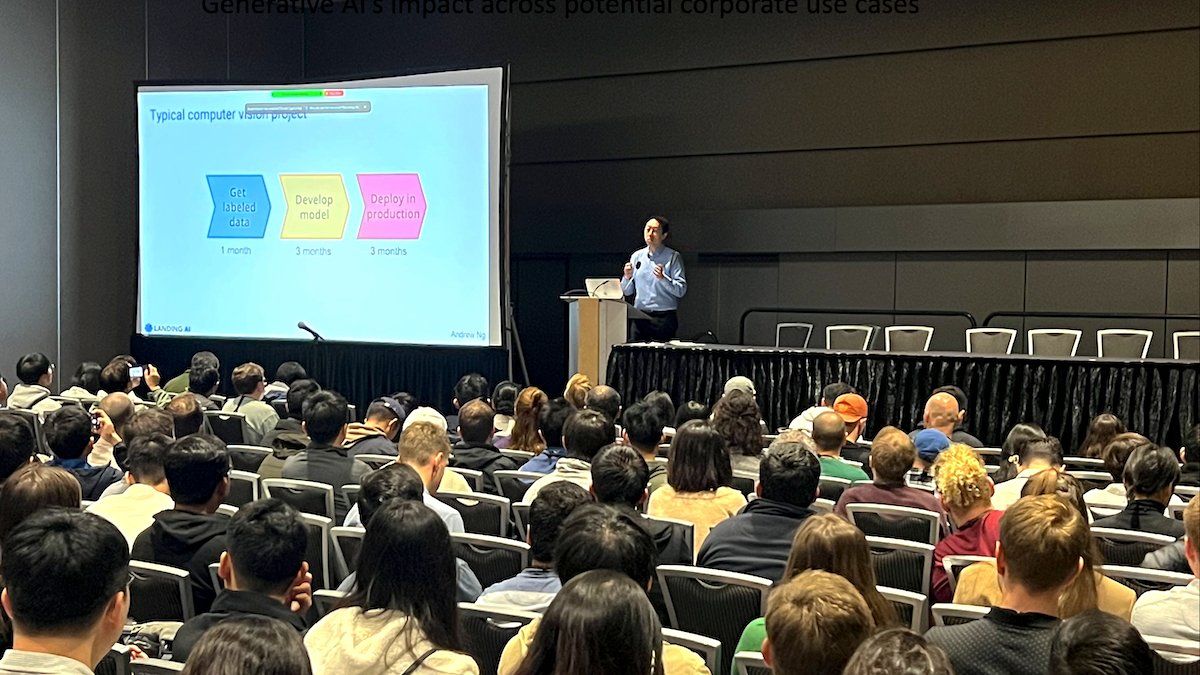
Although it was less pronounced than excitement around the topics above, I also noticed increased interest in multimodal models. Specifically, given that a transformer can convert either an image or a piece of text into a sequence of tokens, you can feed both types of tokens into the same transformer model to have it process inputs that include both images and text. Many teams are exploring architectures like this.
Lastly, even though the roadmap to self-driving cars has been longer than many people expected, there remains a lot of research in this area. I think the rise of large, pretrained transformers will help kickstart breakthroughs in self-driving.
I also spoke at the CVPR conference’s workshop on Computer Vision in the Wild about Landing AI’s work on making computer vision easy, with visual prompting as a key component. (Thank you Jianwei Yang, Jianfeng Gao, and the other organizers for inviting me!) After my presentation, speaking with many users of computer vision, it struck me that there’s still a gap between the problems studied/benchmarks used in academic research and commercial practice. For example, test sets are more important in academic research than in practical applications; I will write more about this topic in the future.
To everyone I met in person at CVPR: Thank you! Meeting so many people made this trip a real highlight for me.
Keep learning!
Andrew
News
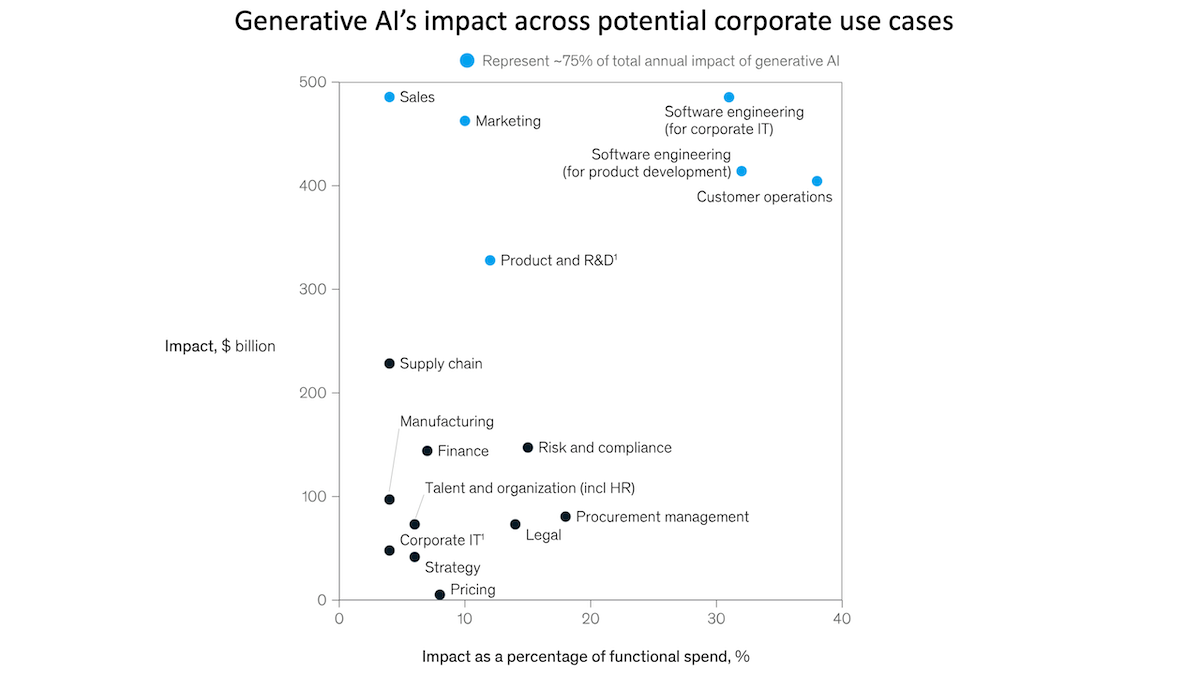
Economic Forecast: GenAI Boom
Generative AI could add between $2.6 trillion and $4.4 trillion to the global economy annually (roughly 2 percent to 4 percent of the world’s combined gross domestic product this year), according to a new report.
What's new: The management consultancy McKinsey projected generative AI’s impacts on productivity, automation, and the workforce in a new report.
How it works: The authors examined adoption scenarios between 2040 and 2060 and their effect on labor productivity through 2040. They evaluated the business impact of generative AI use cases — for instance, large language models applied to customer service — and estimated the economic value those cases would create if they were applied globally. They also assessed the technology’s potential to automate tasks in roughly 850 occupations based on an occupation’s sensory, cognitive, physical, language, and social requirements.
- The high-tech sector is poised to receive the biggest economic boost, as generative AI, if universally adopted, could add between 4.8 to 9.3 percent to its current value. Banking, education, pharmaceuticals, and telecommunications also could experience a large impact, boosting each sector’s value by 2 to 5 percent.
- Four sets of activities — sales and marketing, software engineering, customer operations, and product research and development — represent 75 percent of total potential economic gains.
- In a survey of eight countries that include both developed and developing economies, the authors found that generative AI is likely to automate tasks in relatively high-paying jobs such as software engineering and product development. It will automate the most tasks in jobs that pay in the highest or second-highest income quintiles.
- Generative AI could automate 50 percent of all work tasks between 2030 and 2060. The technology is most likely to automate tasks that require logical reasoning and generating or understanding natural language.
Behind the news: Generative AI’s potential to displace human workers is causing substantial anxiety among the general public. A recent CNBC survey of 8,874 U.S. workers found that 24 percent of respondents were “very worried” or “somewhat worried” that AI would make their jobs obsolete. Respondents were more likely to worry if they were younger (32 percent of respondents of age 18 to 24 compared to 14 percent of those 65 or older), identified as part of a minority (38 percent of Asian respondents, 35 percent of Hispanic respondents, and 32 percent of black respondents versus 19 percent of white respondents), or earned a relatively low income (30 percent of respondents who earn less than $50,000 annually versus 16 percent of those who earn more than $150,000).
Yes, but: As the saying goes, it’s difficult to make predictions, especially about the future. A decade after a 2013 Oxford University study predicted that 47 percent of U.S. jobs were at risk of automation, the U.S. unemployment rate is nearly at record lows. A 2022 study found that employment rates have risen in occupations previously believed to be at risk from AI and robotics.
Why it matters: Generative AI already is having a noticeable effect on venture investments. This analysis indicates that current changes may herald disruptive impacts to come.
We're thinking: Prospective economic gains are good news, but they should be considered in a broader context. We see a real risk that AI may become so good at automating human work that many people will find themselves unable to generate substantial economic value. The best path forward is to democratize the technology so everyone can benefit and make sensible decisions together.
Why it matters: Generative AI already is having a noticeable effect on venture investments. This analysis indicates that current changes may herald disruptive impacts to come.
We're thinking: Prospective economic gains are good news, but they should be considered in a broader context. We see a real risk that AI may become so good at automating human work that many people will find themselves unable to generate substantial economic value. The best path forward is to democratize the technology so everyone can benefit and make sensible decisions together.
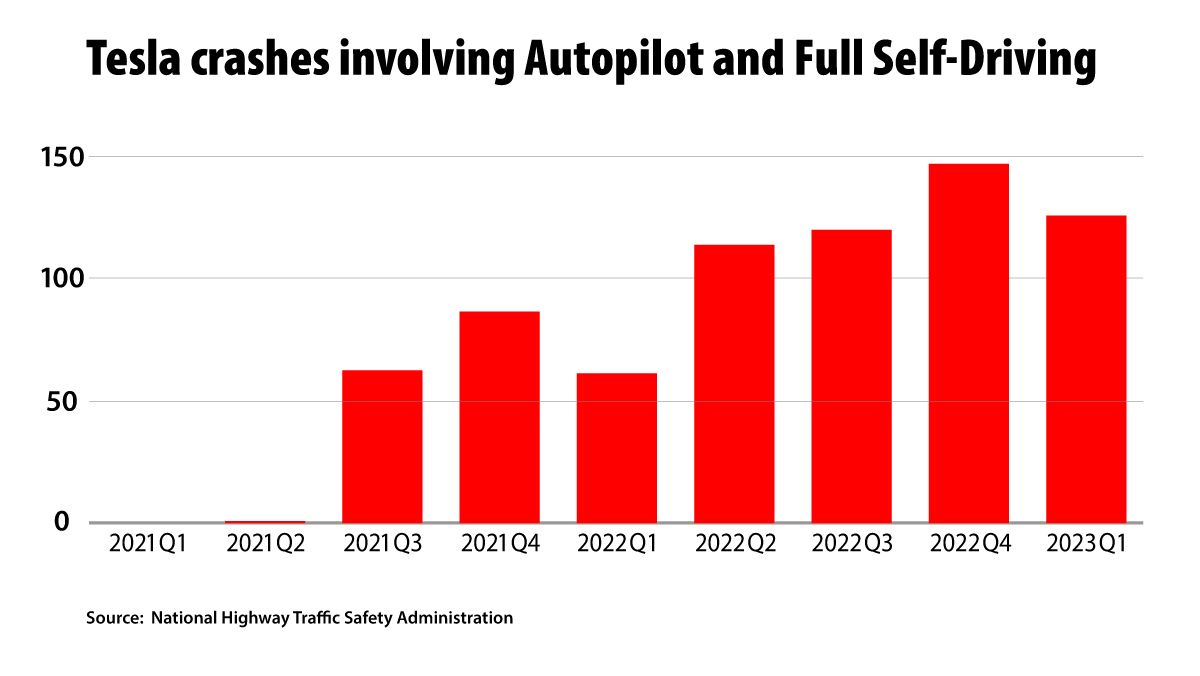
More Tesla Crashes
Tesla cars operating semi-autonomously have had many more collisions than previously reported, government data shows.
What's new: Tesla vehicles operating in the so-called Autopilot or Full Self-Driving mode were involved in 736 U.S. crashes between sometime in 2019 and May 2023, according to data gathered by the United States National Highway Traffic Safety Administration (NHTSA), The Washington Post reported. Earlier data showed that Teslas had been involved in 273 reported crashes between July 2021 and July 2022. The latest data is available at the bottom of this link.
How it works: Tesla offers two semi-autonomous driving modes.
- Autopilot, a standard feature since 2015 that’s currently installed in more than 800,000 vehicles, enables Tesla vehicles to keep themselves in the center of their lane, change lanes, and enter and exit parking spots autonomously.
- What the company calls Full Self-Driving is an optional upgrade that enables Teslas to drive themselves between destinations and automatically brake at intersections and hazards. All Teslas manufactured since 2019 are equipped with the hardware to support this mode, which can be activated for $15,000 or around $99 per month.
The crashes: The NHTSA data is difficult to interpret, since it omits crucial variables such as miles driven and which of Tesla’s two modes was involved in any given crash. Moreover, the earlier and recent crash tallies are difficult to compare due to the difference in their time frames.
- Two-thirds of reported incidents occurred since June 2022, and 17 resulted in fatalities.
- The highest quarterly total roughly coincides with Tesla’s decision in November 2022 to stop restricting Full Self-Driving to Tesla owners whose driving scored highly on certain safety metrics and offer the upgrade to all customers — about 400,000 drivers — regardless of their safety score.
- Tesla’s own safety report, unlike the NHTSA data, tallies accidents per mile driven, comparing driving with Autopilot engaged, driving without Autopilot, and the U.S. average. It shows that, during the period covered by the NHTSA report, Teslas driving with Autopilot engaged experienced far fewer crashes per mile driven than both Teslas without Autopilot and the U.S. average. The Tesla report does not include crashes while driving with Full Self-Driving engaged.
Behind the news: Since August 2021, NHTSA has opened numerous probes into Tesla’s autonomous systems. Repeated incidents under investigation include abrupt braking in the path of following vehicles; collisions with emergency vehicles; and allegations that, in multiple crashes, Autopilot disengaged less than a second before the collision, giving drivers little time to react.
Why it matters: Tesla has claimed repeatedly that its autonomous driving capability is far safer than human drivers. Without knowing which mode was involved in how many crashes over how many miles, that claim is impossible to verify. Meanwhile, there are indications that Tesla may have deliberately misled the public about its self-driving capabilities in the past.
We're thinking: Engineers who work on systems that are critical to safety have a special responsibility to make sure their products are safe and well understood by users. We urge Tesla engineers to shed more light on the performance of these potentially life-threatening systems.
A MESSAGE FROM LANDING AI
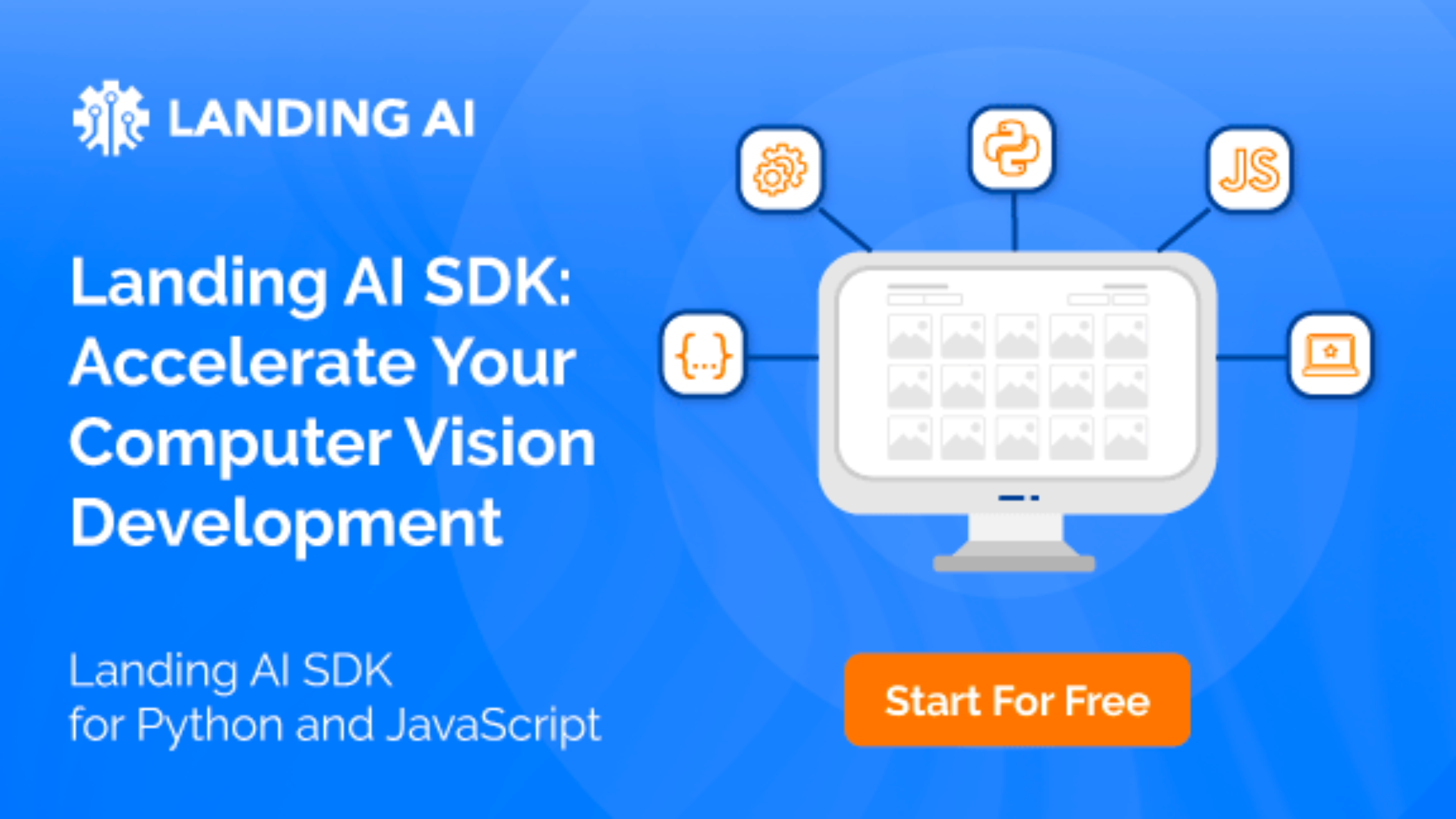
Want to build computer vision into your applications? Train a model in LandingLens (get started for free), then use the Landing AI SDK to easily build custom applications that leverage your model!
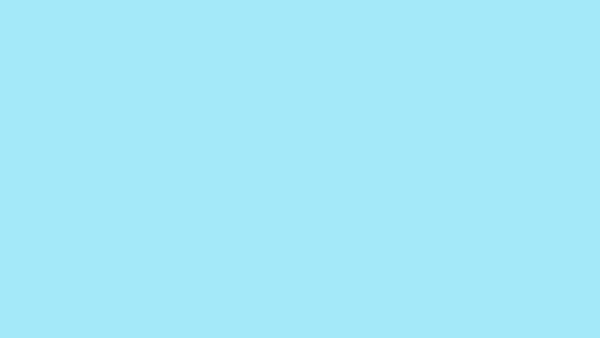
Lawyers: Beware LLMs
A United States federal judge threw ChatGPT’s legal research out of court.
What’s new: An attorney who used ChatGPT to generate a legal brief faces disciplinary action after opposing lawyers discovered that the brief referred to fictional cases and quotations invented by the chatbot, The New York Times reported.
Citation situation: The lawyer, Steven A. Schwartz, was assisting in a personal injury lawsuit on the plaintiff’s side in a federal court in New York City. When the defendant appealed to have the case dismissed, Schwartz countered with a brief based on results from a ChatGPT query.
- Schwartz asked the model to find similar cases in which rulings had favored his client’s argument. It cited six cases and offered quotations from the rulings. He asked the model to verify that the cases were real, and it responded with variations of “The case does indeed exist and can be found in legal research databases such as Westlaw and LexisNexis.”
- He and his co-attorneys filed the resulting brief to the court. The defendant’s lawyers, upon reviewing the document, notified the judge that they were unable to find further information about any of the cases.
- When the judge sought clarification, Schwartz filed a statement admitting to the error and expressing regret. He had never used ChatGPT before, he said, and did not know it was unreliable.
- Schwartz and his firm’s lead lawyer on the case face an in-person disciplinary hearing on June 8.
Ripple effects: In the case’s wake of this case, a federal judge in Texas decreed that lawyers in cases before him may use generative AI to write their briefs only if they file paperwork stating that they manually verified the output for accuracy.
Why it matters: Within the AI community, it may be common knowledge that large language models sometimes confidently state falsehoods as though they were true. Among the general public, though, this fact may not be so well understood. Schwartz’s mishap is a painfully public demonstration of what can happen when people trust such models to supply facts.
We’re thinking: People outside the AI community might reasonably assume that the technology is qualified to assist in legal research.  After all, in April, GPT-4, the large language model behind the most powerful version of ChatGPT, reportedly ranked in the 90th percentile on a U.S. bar exam. (A recent reappraisal revised GPT-4’s score downward to between the 68th and 48th percentiles.) This goes to show that AI performance on these tests doesn’t necessarily map well to human performance, since any junior law student would know not to invent cases. There’s important work to be done to apply LLMs to legal work. Meanwhile, we urge researchers who are testing LLMs’ ability to meet real-world qualifications to resist hype when reporting their results.
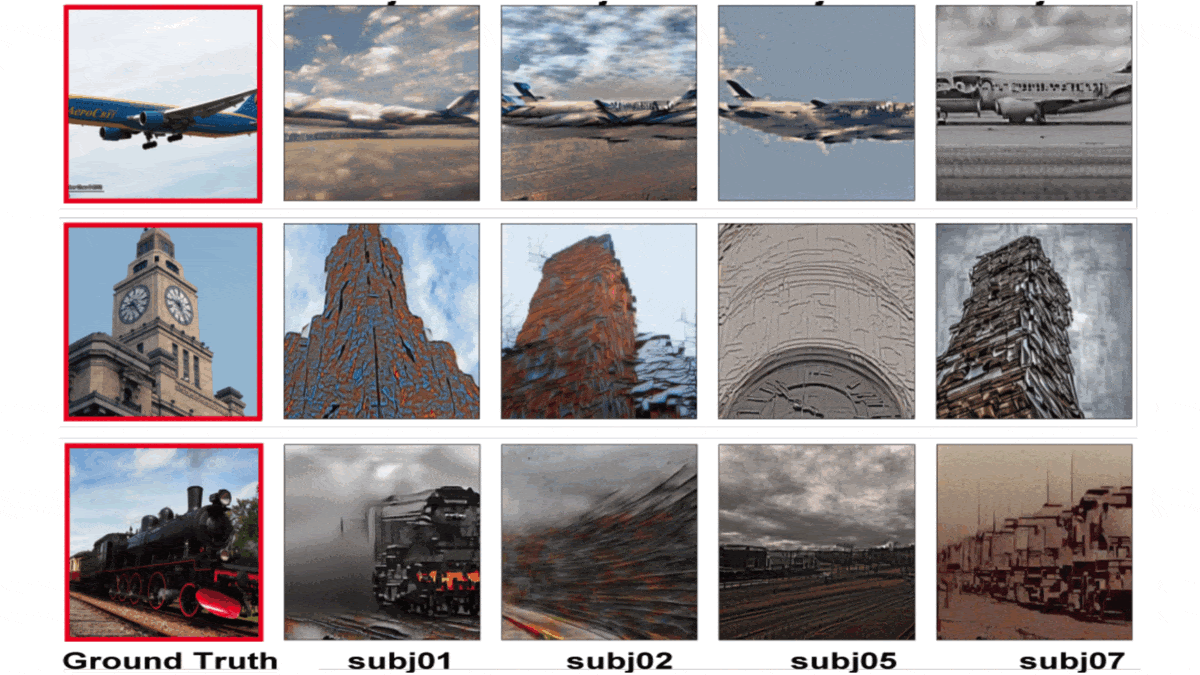
What the Brain Sees
A pretrained text-to-image generator enabled researchers to see — roughly — what other people looked at based on brain scans.
What's new: Yu Takagi and Shinji Nishimoto developed a method that uses Stable Diffusion to reconstruct images viewed by test subjects from scans of their brains that were taken while they were looking at the images.
Diffusion model basics: During training, a text-to-image generator based on diffusion takes a noisy image and a text description. A model embeds the description, and a diffusion model learns to use the embedding to remove the noise in successive steps. At inference, the system starts with pure noise and a text description, and iteratively removes noise according to the text to generate an image. A variant known as a latent diffusion model saves computation by embedding the image as well and removing noise from noisy versions of the embedding instead of a noisy image.
Key insight: Stable Diffusion, like other latent diffusion text-to-image generators, uses separate embeddings of corresponding images and text descriptions to generate an image. In an analogous way, the region of the human brain that processes input from the eyes can be divided into areas that process the input’s purely sensory and semantic aspects respectively. In brain scans produced by functional magnetic resonance imaging (fMRI), which depicts cortical blood oxygenation and thus indicates neuron activity, these areas can be embedded separately to substitute for the usual image and text embeddings. Given these embeddings, Stable Diffusion can generate an image similar to what a person was looking at when their brain was scanned.
How it works: The authors trained a simple system to produce input for Stable Diffusion based on fMRI. They trained a separate version of the system for each of four subjects whose brains were scanned as they looked at 10,000 images of natural scenes.
- Given a photo with associated text, Stable Diffusion’s encoders separately embedded the photo and the text.
- The authors trained two linear regression models. One learned to reproduce Stable Diffusion’s image embedding from the part of the fMRI scan that corresponds to the brain’s early visual cortex (which detects the orientation of objects), and the other learned to reproduce Stable Diffusion’s text embedding from the part of the fMRI scan that corresponds to the ventral visual cortex (which decides the meaning of objects).
- At inference, given an fMRI scan, the linear regression models produced image and text embeddings. The authors added noise to the image embedding and fed both embeddings to Stable Diffusion, which generated an image.
Results: The authors concluded that their approach differed so much from previous work that quantitative comparisons weren’t helpful. Qualitatively, the generated images for all four subjects depict roughly the same scenes as the ground-truth images, though the details differ. For instance, compared to the ground-truth image of an airplane, the generated images appear to show something airplane-shaped but with oddly shaped windows, a cloudier sky, and blurred edges.
Why it matters: Previous efforts to reproduce visual images from brain scans required training a large neural network. In this case, the authors trained a pair of simple linear models and used a large pretrained model to do the heavy lifting.
We’re thinking: The generated images from models trained on brain scans of different subjects showed different details. The authors suggest that this disagreement may have arisen from differences in the subjects’ perceptions or differences in data quality. On the contrary, they may relate to the noise added during image generation.
Data Points
What stands in the way of Nvidia competitors? Nvidia
Despite attempts by companies like AMD to introduce new AI chips, Nvidia's market presence and technological advantage have widened the gap in sales and performance. (Financial Times)
Google Shopping launched AI features
Newly available to U.S. users: A generative AI-powered virtual try-on feature and the option to refine a product search with visual matching. (The Verge)
European Parliament approved the AI Act
The vote marks a milestone in an ongoing process. Negotiations among EU representatives will determine the final version of the proposal, which is expected to be finalized by the end of the year. (The New York Times)
EU launch of Google's Bard delayed over privacy issues
The chatbot is available in 180 new countries except for EU nations due to the company's failure to address privacy concerns raised by the Irish Data Protection Commission. (The Verge)
Paul McCartney is using AI to produce new Beatles record
During an interview, the singer said that his team is using AI tools to extract John Lennon's voice from an old demo and incorporate it into an upcoming track. (The Verge)
Adobe enhances Illustrator with generative AI
A new Generative Recolor feature is available in beta. It allows users to use text prompts to change colors and fonts in graphics. (Forbes)
OpenAI sued for defamation after ChatGPT fabricated accusations
A radio host filed a lawsuit against OpenAI in a Georgia state super court. He alleges that the chatbot made false allegations against him when a journalist used it to summarize a different, federal court case. (The Verge)
Media giants discussing potential protections against generative AI
News organizations including The New York Times and NBC News are holding talks about safeguards and rules to protect their content against aggregation and misrepresentation by generative AI tools. (CNBC)
Artist used Stable Diffusion to generate illustrations that contain hidden QR codes
The artist trained custom Stable Diffusion ControlNet models to develop functional QR codes that masquerade as anime and other Asian art styles. (Ars Technica)
Japanese city adopted ChatGPT in administrative operations
After a successful one-month trial, Yokosuka will start using the tool to summarize meetings, edit documents, and other tasks, aiming to improve efficiency and shorten business hours. (Japan Times)